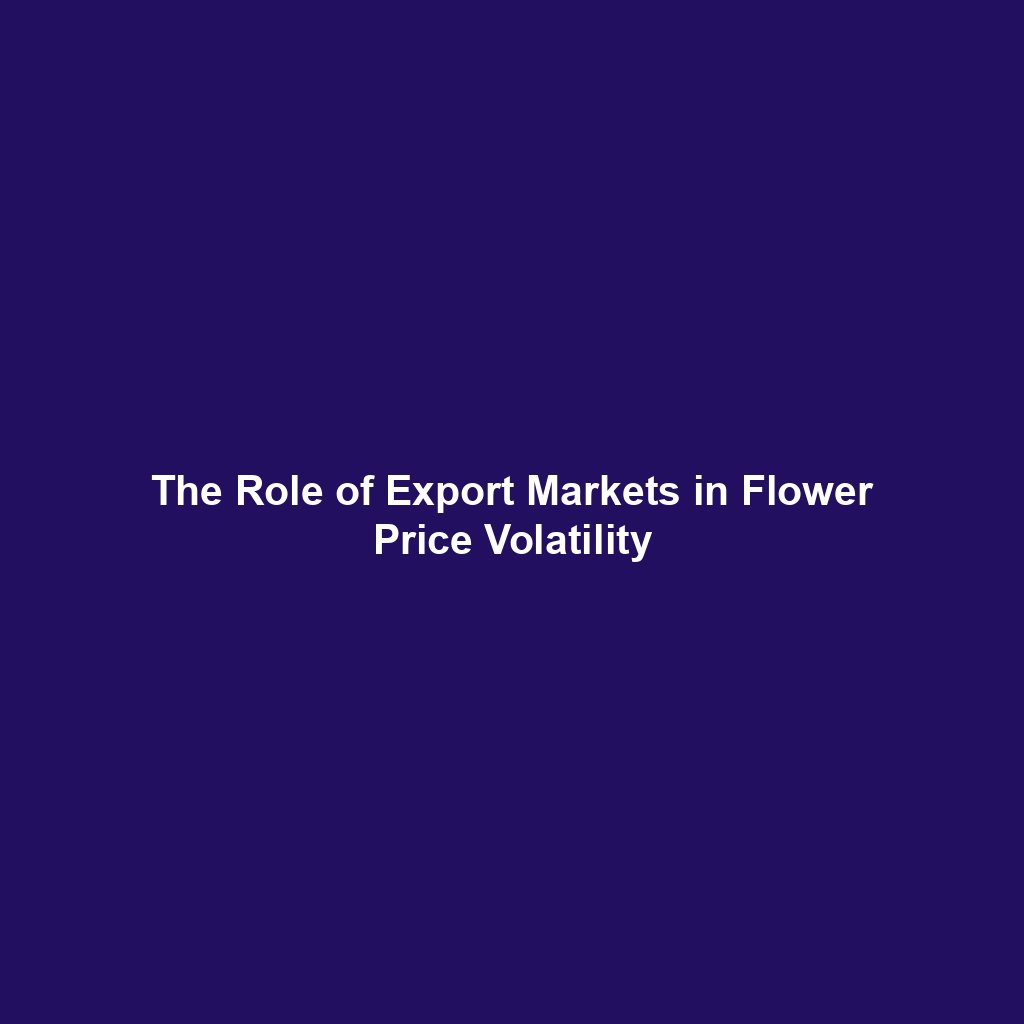
Agriculture and agricultural price analysis are critical components of the global economy, influencing food security, trade balances, and rural development. This article delves into the complexities of agricultural markets, focusing on the factors that drive price volatility and the methodologies used to analyze these fluctuations. By understanding these dynamics, stakeholders can make more informed decisions, from farmers planning their crop cycles to policymakers crafting agricultural policies.
Chapter 1: Understanding Agricultural Markets
The Structure of Agricultural Markets
Agricultural markets are characterized by a unique set of features that distinguish them from other types of markets. These include seasonality, perishability, and the significant influence of weather conditions. The structure of these markets can be broadly categorized into three segments: input markets, production markets, and output markets.
- Input Markets: These markets deal with the supply of essential inputs such as seeds, fertilizers, pesticides, and machinery. The prices in these markets can significantly impact the cost of production and, consequently, the final price of agricultural products.
- Production Markets: This segment involves the actual cultivation and harvesting of crops. Factors such as land availability, labor costs, and technological advancements play a crucial role in determining the efficiency and output of agricultural production.
- Output Markets: These markets handle the distribution and sale of agricultural products. They are influenced by factors such as demand and supply dynamics, transportation costs, and market access.
Factors Influencing Agricultural Prices
Agricultural prices are influenced by a myriad of factors, both internal and external. Understanding these factors is essential for effective price analysis and forecasting.
- Supply and Demand: The most fundamental factor affecting agricultural prices is the balance between supply and demand. An oversupply of a particular crop can lead to a price drop, while a shortage can cause prices to spike.
- Weather Conditions: Weather plays a pivotal role in agricultural production. Droughts, floods, and other extreme weather events can drastically affect crop yields, leading to price volatility.
- Government Policies: Subsidies, tariffs, and trade restrictions can significantly impact agricultural prices. For instance, export bans on certain crops can lead to a surplus in the domestic market, driving prices down.
- Global Markets: The interconnectedness of global markets means that events in one part of the world can have ripple effects elsewhere. For example, a poor harvest in a major wheat-producing country can lead to higher global wheat prices.
- Technological Advancements: Innovations in agricultural technology, such as genetically modified crops and precision farming, can increase productivity and affect prices.
Chapter 2: Agricultural Price Analysis
Methods of Price Analysis
Price analysis in agriculture involves various methodologies, each with its strengths and limitations. These methods can be broadly categorized into qualitative and quantitative approaches.
- Qualitative Analysis: This approach involves the use of expert opinions, market reports, and historical data to understand price trends. While it provides valuable insights, it is often subjective and may not capture all the complexities of the market.
- Quantitative Analysis: This method uses statistical and econometric models to analyze price data. Techniques such as time-series analysis, regression models, and machine learning algorithms can provide more objective and precise forecasts.
Time-Series Analysis
Time-series analysis is a popular method for analyzing agricultural prices. It involves the study of price data over time to identify trends, cycles, and seasonal patterns. Key techniques in time-series analysis include:
- Moving Averages: This technique smooths out short-term fluctuations to highlight longer-term trends. It is particularly useful for identifying seasonal patterns in agricultural prices.
- Autoregressive Integrated Moving Average (ARIMA): ARIMA models are widely used for forecasting agricultural prices. They combine autoregression, differencing, and moving averages to capture the underlying patterns in the data.
- Seasonal Decomposition of Time Series (STL): STL is a robust method for decomposing time-series data into trend, seasonal, and residual components. It is particularly useful for understanding the seasonal variations in agricultural prices.
Regression Analysis
Regression analysis is another powerful tool for agricultural price analysis. It involves the use of statistical techniques to model the relationship between a dependent variable (e.g., agricultural prices) and one or more independent variables (e.g., weather conditions, input costs). Key types of regression analysis include:
- Linear Regression: This is the simplest form of regression analysis, where the relationship between the dependent and independent variables is assumed to be linear. It is useful for identifying the impact of individual factors on agricultural prices.
- Multiple Regression: This technique extends linear regression by incorporating multiple independent variables. It provides a more comprehensive understanding of the factors influencing agricultural prices.
- Logistic Regression: This method is used when the dependent variable is categorical (e.g., price increase or decrease). It is useful for predicting the probability of different price outcomes based on various factors.
Machine Learning Algorithms
With the advent of big data and advanced computing technologies, machine learning algorithms have become increasingly popular for agricultural price analysis. These algorithms can handle large datasets and capture complex, non-linear relationships between variables. Key machine learning techniques include:
- Random Forest: This ensemble learning method combines multiple decision trees to improve prediction accuracy. It is particularly useful for handling high-dimensional data and capturing non-linear relationships.
- Support Vector Machines (SVM): SVMs are powerful classification and regression techniques that can handle both linear and non-linear data. They are useful for identifying patterns and making accurate price forecasts.
- Neural Networks: These algorithms are inspired by the human brain and can model complex, non-linear relationships. They are particularly effective for capturing intricate patterns in agricultural price data.
Challenges in Agricultural Price Analysis
Despite the availability of advanced analytical techniques, agricultural price analysis faces several challenges. These include:
- Data Quality: The accuracy of price forecasts depends on the quality of the data used. In many cases, agricultural data may be incomplete, outdated, or inconsistent, making it difficult to obtain reliable results.
- Market Volatility: Agricultural markets are inherently volatile, influenced by a multitude of unpredictable factors. This makes it challenging to develop accurate and robust price models.
- Complex Interactions: The interactions between different factors influencing agricultural prices are often complex and non-linear. Capturing these interactions requires sophisticated modeling techniques and a deep understanding of the market dynamics.
- Policy Changes: Government policies can have a significant impact on agricultural prices. Sudden changes in policies, such as the introduction of new subsidies or trade restrictions, can disrupt market dynamics and make it difficult to predict price movements.
Conclusion
Agriculture and agricultural price analysis are vital for ensuring food security, economic stability, and sustainable development. By understanding the structure of agricultural markets and the factors influencing price volatility, stakeholders can make more informed decisions. Advanced analytical techniques, such as time-series analysis, regression models, and machine learning algorithms, provide valuable tools for analyzing and forecasting agricultural prices. However, challenges such as data quality, market volatility, and complex interactions must be addressed to improve the accuracy and reliability of price forecasts. As the global agricultural landscape continues to evolve, ongoing research and innovation in price analysis will be essential for navigating the complexities of agricultural markets and ensuring a stable and prosperous future for all.